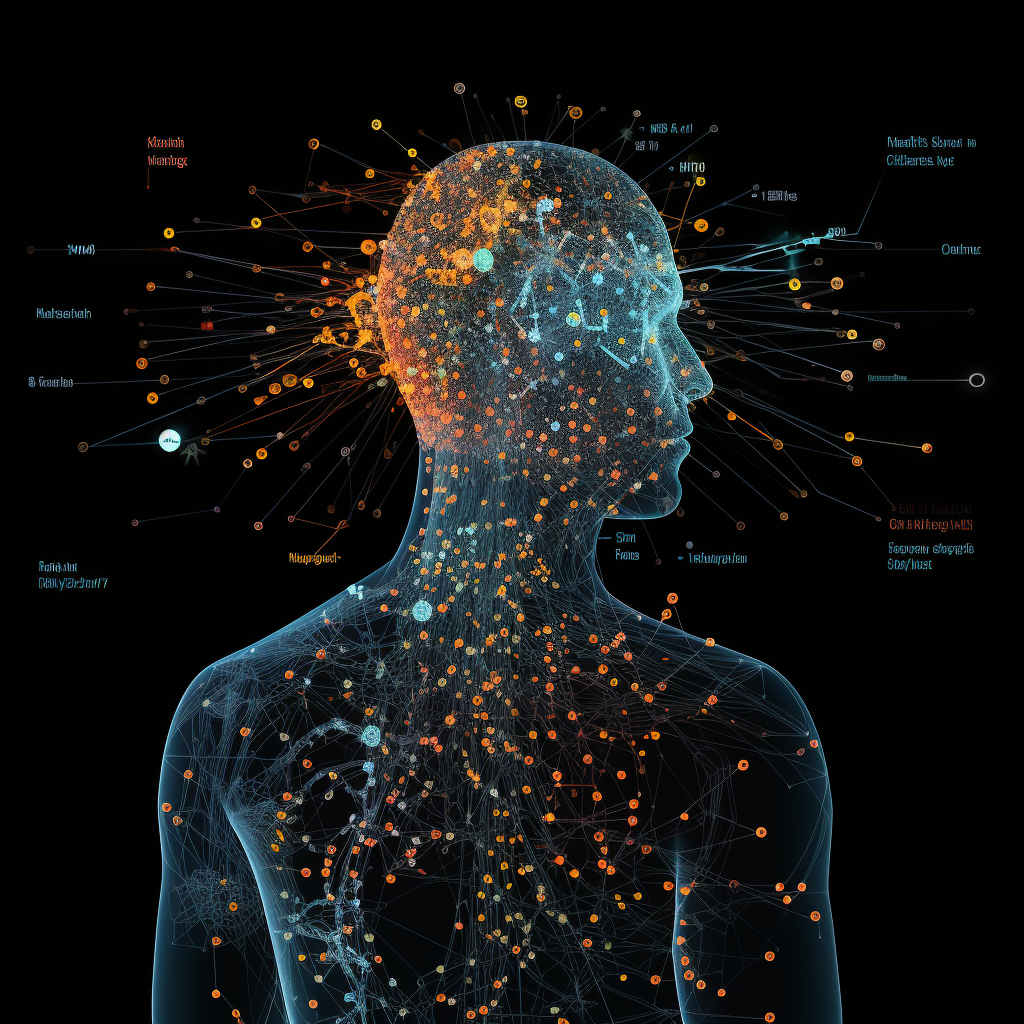
Immune-checkpoint blockade therapies have revolutionized the treatment of metastatic melanoma in recent years. However, these therapies do not provide a long-lasting response for many patients. Indeed, 50% of patients succumb to metastatic disease within five years of diagnosis, with responses to immunotherapies even varying between different metastases within a single patient. The tumor microenvironment, specifically immune cells within it, plays a pivotal role in shaping tumor progression. Despite this, we still lack biomarkers to guide therapy and predict response to specific immunotherapies.
In this project, Leeat Keren's group is integrating innovative imaging technology with advanced AI tools to decipher these images and identify predictive biomarkers for treatment success or resistance. The project employs a state-of-the-art high-dimensional imaging technology known as MIBI-TOF (Multiplexed Ion Beam Imaging by Time of Flight). This technology is capable of high-throughput imaging of dozens of proteins at sub-cellular resolution, allowing for profiling the tumor immune microenvironment in over 100 melanoma patients treated at Hadassah Medical Center.
For each tumor slice, MIBI-TOF generates a stack of dozens of images. Each image portrays the intensity and location of a different protein in the tissue. AI tools, such as convolutional neural networks, autoencoders, and transformers, allow researchers to combine these individual images into descriptors of tumor cells and their surrounding microenvironment. This assists in understanding which cells are present in the tumor microenvironment, their functions, and their interactions with each other. This in-depth understanding is then correlated with patient survival and response to therapy. The ultimate goal is to develop a clinically applicable method for guiding patient-specific selection of therapies from a rapidly expanding arsenal of cancer immunotherapies.