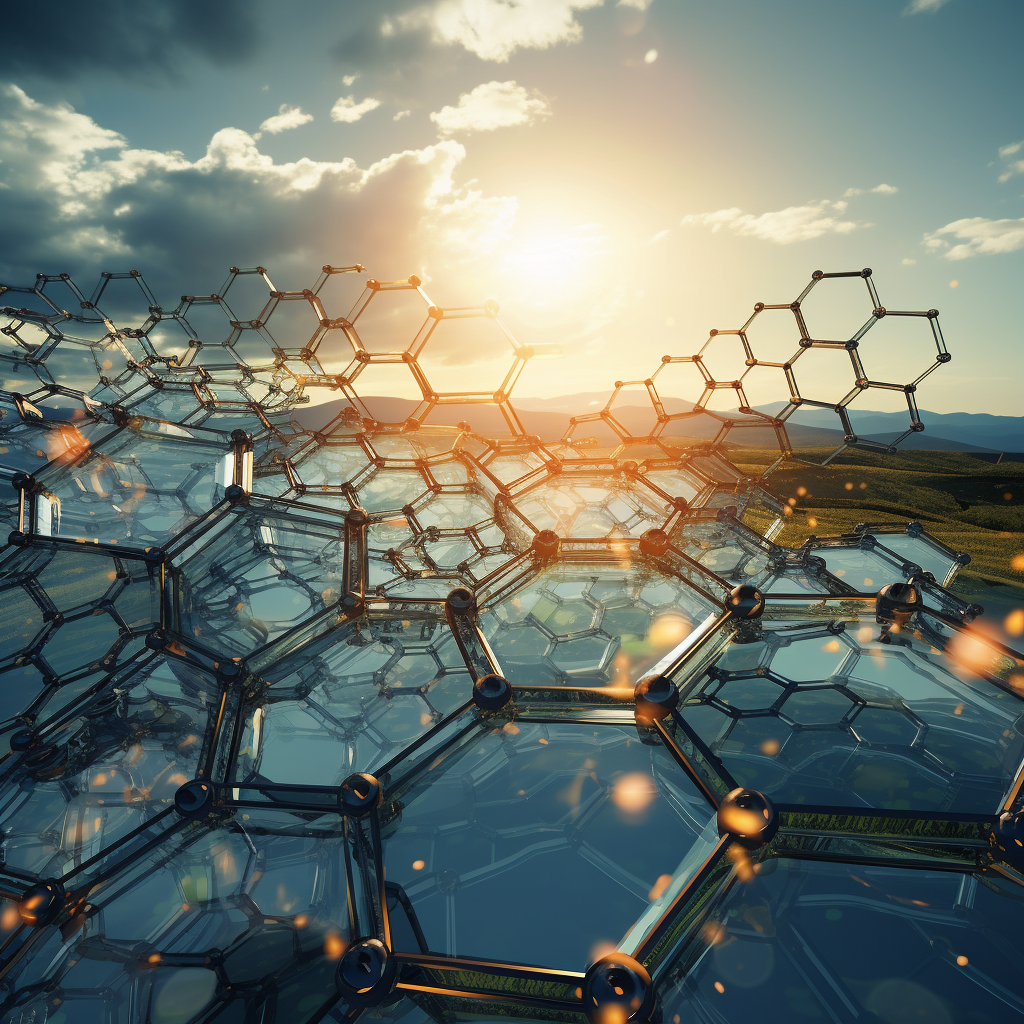
The dynamical mechanisms involved in light-matter interaction in materials, dominated by energetically excited quantum states, are notably complex. These complexities present a significant challenge for traditional scientific approaches. To address this, materials science groups at the Weizmann Institute are working on establishing a new theoretical learning algorithm. They are applying AI-based and machine learning approaches to evaluate and understand the outcomes of these intricate particle-particle interactions. This strategy will not only provide insights into the quantum behavior of materials when exposed to sunlight but also offers valuable information for optimizing material structures to enhance these interactions.
Diving into machine learning methods, the Kronik group studies structure dynamics of emerging systems in solar energy conversion, such as perovskites and molecular crystals—two highly important materials that have yet to be optimized in terms of their structure.
The Refaely-Abramson group aims to bridge the gap between quantum theory of particle dynamics and practical applications, such as in the development of more efficient photovoltaic cells and material-based quantum information storage.
By leveraging AI, their approach has the potential to revolutionize our understanding of light-matter interactions in realistic materials at the quantum level, paving the way for the design of materials with bespoke quantum properties.