About the Project
Denoising MIBI images with AI is a vital endeavor that will improve our understanding of cellular function and cancer development.
MIBI-TOF is a cutting-edge technology that facilitates high-dimensional single-cell imaging of clinical human specimens. By staining tissue sections with numerous metal-labeled antibodies and employing secondary ionization mass spectrometry, MIBI-TOF produces multi-dimensional images that reveal sub-cellular expression and localization for dozens of distinct proteins in situ.
However, MIBI-TOF data noise obscures critical information and impedes accurate analysis. Current manual denoising methods are time-consuming, labor intensive, and subjective, which introduces variance between different individuals and instances. To address these challenges, the research team at the AI Hub is exploring innovative denoising approaches inspired by recent advancements in machine learning. Traditionally, denoising methods require both clean and noisy versions of images for training. The team is investigating the Noise2Void technique, which only necessitates a single noisy image by leveraging blind spot analysis to infer the clean data. By training the system to distinguish between noise and signal, the denoising process becomes more efficient and less resource-intensive.
Research conducted by Leeat Keren indicates the importance of understanding the cumulative behavior of cells. Her work in Triple Negative Breast Cancer showed that patients in which the immune cells are able to generate local hubs live longer than patients in which the immune cells are dispersed within the tumor.
The application of AI in denoising MIBI images will enable researchers to more effectively analyze imaging data, leading to deeper insights into tissue functionality, particularly in the context of diseases like cancer. Understanding the cumulative behavior of cells through multiplexed imaging holds promise for developing more targeted and effective treatments for various ailments.
The research team is currently collaborating on several angles, such as single channel denoising (supervised vs. self supervised), channel families denoising, and multi channel denoising, harnessing the power of AI-driven automation to optimize MIBI images and advance our understanding of cellular processes. This interdisciplinary project reflects the collaborative efforts between the fields of biology and computational AI at the Weizmann Institute AI Hub.
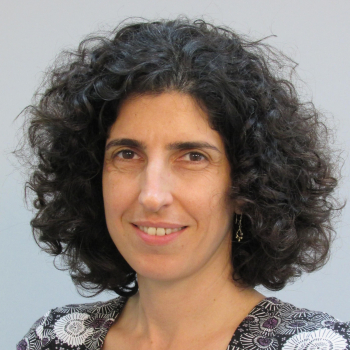
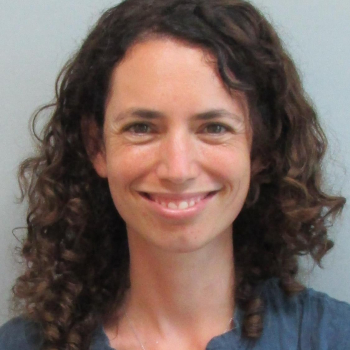