About the Project
Applying AI methods in the field of physics presents significant challenges. While most AI methods offer solutions that resemble a “black box”, when looking to understand the underlying physical mechanisms, causality relations need to be established to elucidate the fundamental principles governing the physical phenomena.
In our project at the AI-Hub, we introduce an approach that enables AI explainability in physical processes using unsupervised learning. We demonstrate our method on organic molecular crystals, where complex dynamics such as wavepacket propagation are initiated by an optical excitation. The dynamics in these types of materials involve convoluted spatial correlations that evolve over time, making them of great interest for applications in the fields of quantum computing and quantum information. The ability to resolve structural dependencies of these processes is necessary to generate design principles and optimize material functionality. Yet, achieving this goal remains a significant challenge due to the coordinated participation of hundreds of different particles.
In view of these challenges, resolving these processes within the framework of AI is highly intriguing. More specifically, unsupervised AI methods, which specialize in arranging such a wealth of physical information into clusters sharing similar properties, could shed light on the mechanisms involved.
We developed a novel scheme based on a singular value decomposition (SVD) that transforms the original coordinates, defined by the input data, into a new coordinate domain that accounts for and preserves the internal correlations in both time and space. We exploit the coupling between each pair of left and right singular vectors to reconstruct dynamical features that allow direct recognition of the relaxation and dephasing mechanisms dominating the underlying dynamics. We further demonstrate how the contribution of these features varies with changes in the initial conditions determined by the shape of the excited pulse, opening the way for material-based coherent control. Finally, we construct entangled virtual features, used to study the phase relations dominating the combined dephasing components. By this, our method introduces a step-by-step analysis of the involved convoluted mechanisms without reducing information from the input data set, thereby allowing for the explainability of the underlying dynamics.
As is the case with other AI methods, our approach is general and can be applied to unravel complex interconnections in fields such as biology and environmental sciences, where multi-dimensional correlations play a crucial role.
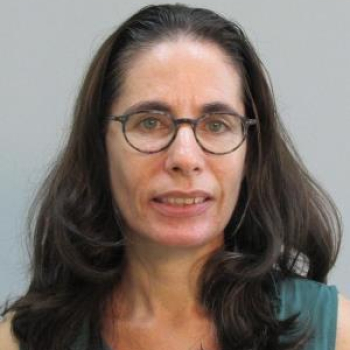
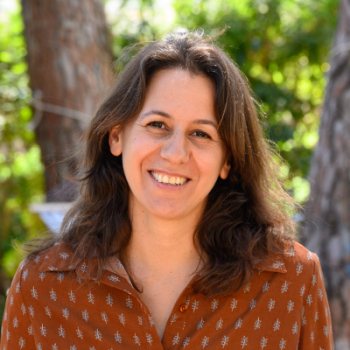